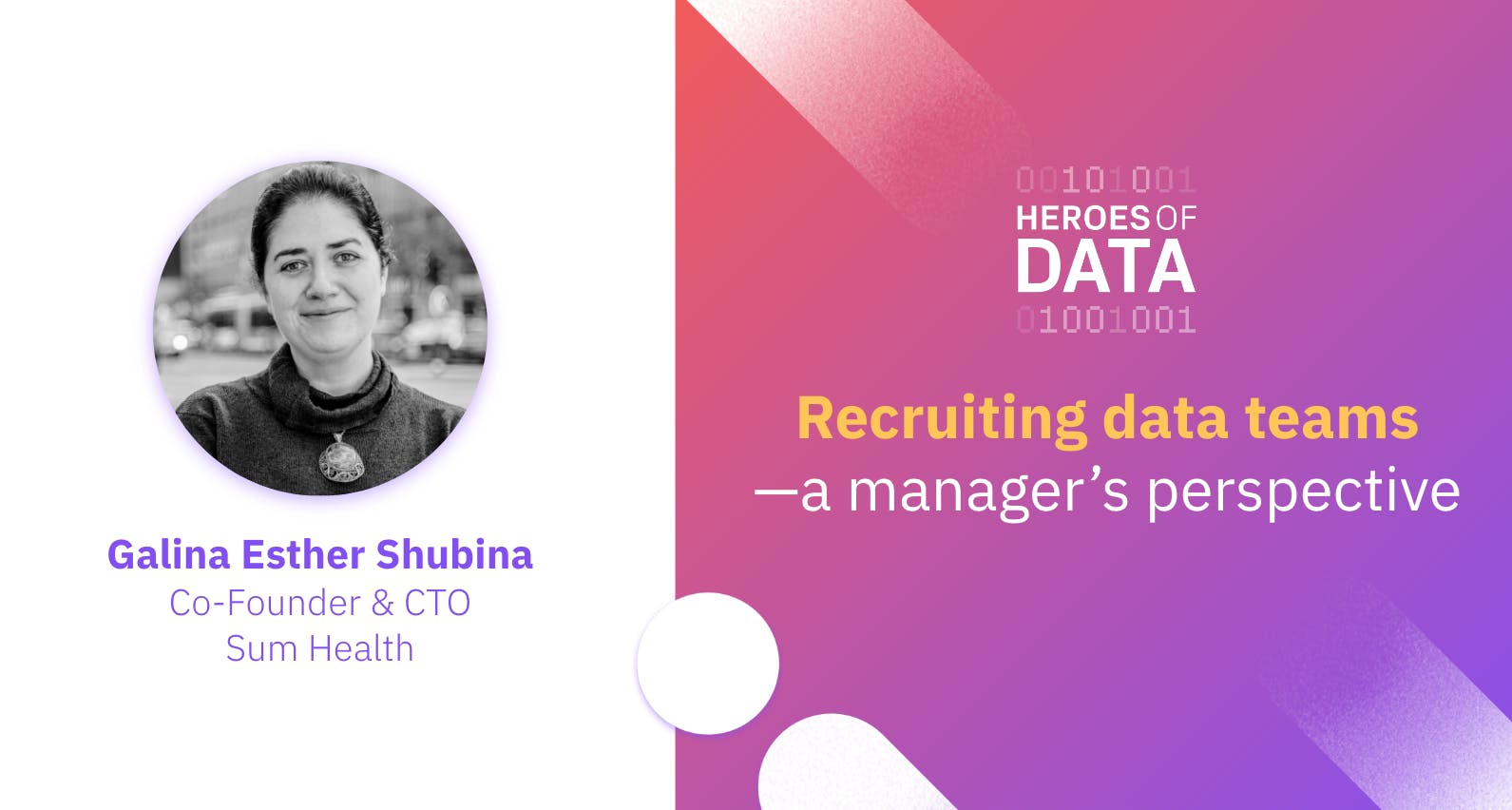
Galina is one of the most senior data professionals in the Nordics
Galina has an impressive story and resume, so it requires some effort to keep this introduction brief–but here goes nothing:
Galina was born in the Soviet Union. In the early 90s, she moved to the US with her family to live on the East Coast, where she obtained a Master’s degree in computer science at Brown University. In 2004, Galina moved to Silicon Valley to join this little company called Google (yes, it was little at the time) and started in their data science team. This was about three years ahead of when data science was an established term, when these data scientists did their own data engineering, their own data analysis, and presented all data visualizations to executives on their own. Today’s generation of businesses offers far more specialized data-related roles of various titles, and the understanding of these roles is getting richer each year. We have come a long way since Galina’s early days in Silicon Valley.
From Google to Northvolt to co-founder
Fast forward a bit, Galina moved to Sweden with her Swedish partner, and, after having worked at Google for 10 years, joined Schibsted to build their Data and Analytics Team. She then started her own consulting firm, Gradient Descent, which helps companies figure out what to do with their data. In 2018, she built a Data and AI team at Northvolt from scratch (dubbed Sweden’s #1 startup in 2022 by LinkedIn). Now, she is the co-founder and CTO of a health tech startup called SumHealth. SumHealth’s goal is to utilize data to allow people to find their diagnosis faster by learning from people like them.
On top of this, she also co-founded Women In Data Science AI & ML Sweden in late 2017, to bring more female technologists into the field and help them receive recognition for their work. They currently have more than 1,600 women in their network.
Ok, that was keeping it brief. Suffice to say, Galina has done great things in the data science field for a long time, building data teams for almost two decades now. Let’s dive into her practical tips on recruiting for data teams.
Recruiting data teams takes too long for many companies
At the beginning of Galina’s presentation, she showed a job posting example for a head of data, with a long list of requirements. Looking at each bullet point individually, they are realistic, but for a candidate to have them all, less so. A company going out with a job posting like this is basically saying they are looking for a unicorn.
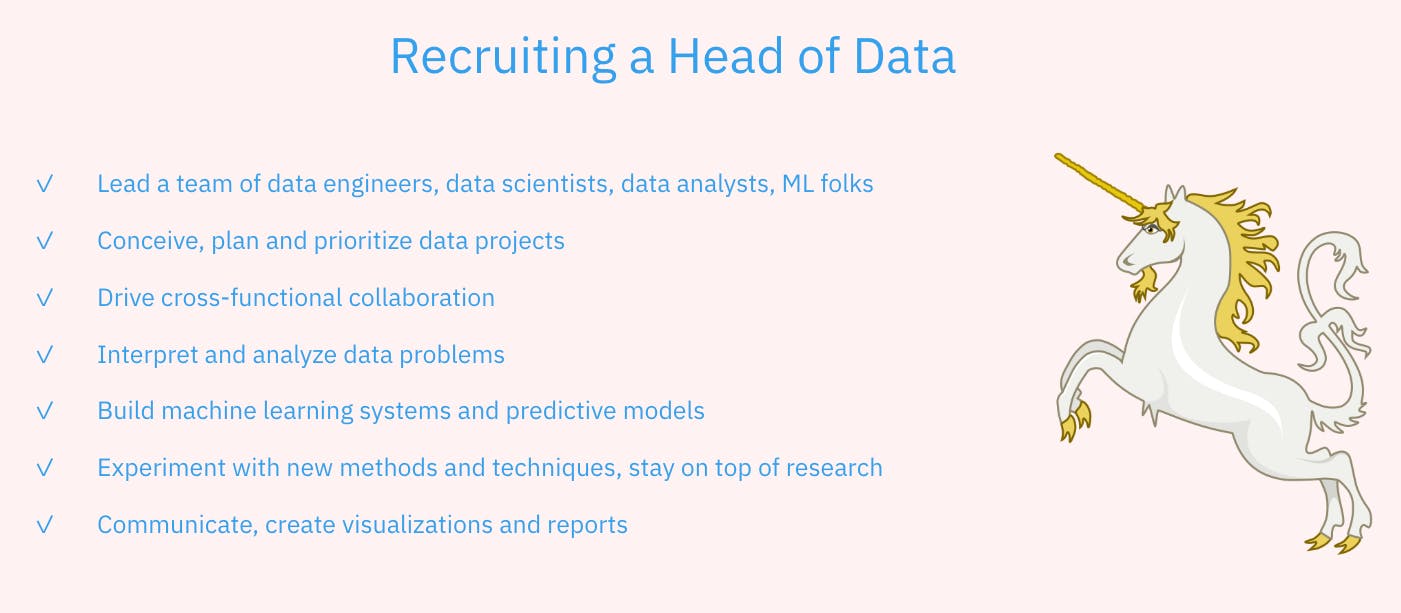
Out of all industries, Information Technology (i.e. including all sub industries such as data, software engineering, cyber security, etc.) has the highest average time to fill an open position. This Swedish study showed an average time-to-fill of 2.6 months for IT roles, from when a job ad is listed to when a candidate has signed an offer. Note that this metric even excluded notice periods, which tend to be three months in Sweden, compared to two weeks in the US.
Of course, many factors may affect the time-to-fill. In some cases, it takes multiple technical interviews and screens before you are through to the final rounds of meeting the hiring manager and your team. In other cases, slow processes and dormant communications are to blame.
Here’s how to make recruiting data teams better for everyone involved
Don’t worry–with a structured and standardized approach to recruiting data teams, you can drastically reduce this time-to-fill as well as improve the overall recruiting experience for both recruiters and candidates. Let’s look at Galina’s four-step model for achieving this: figuring out what your company needs, finding candidates, evaluating candidates, and closing candidates.
1. Figure out what your company needs
What do you want to build with data?
Before you start deciding on what roles you will fill your data team with, it’s crucial to identify what your business needs are, and how data can be leveraged to meet those needs. Galina argues there are generally four big business areas for data teams, illustrated in the image below (you can read more about it here).
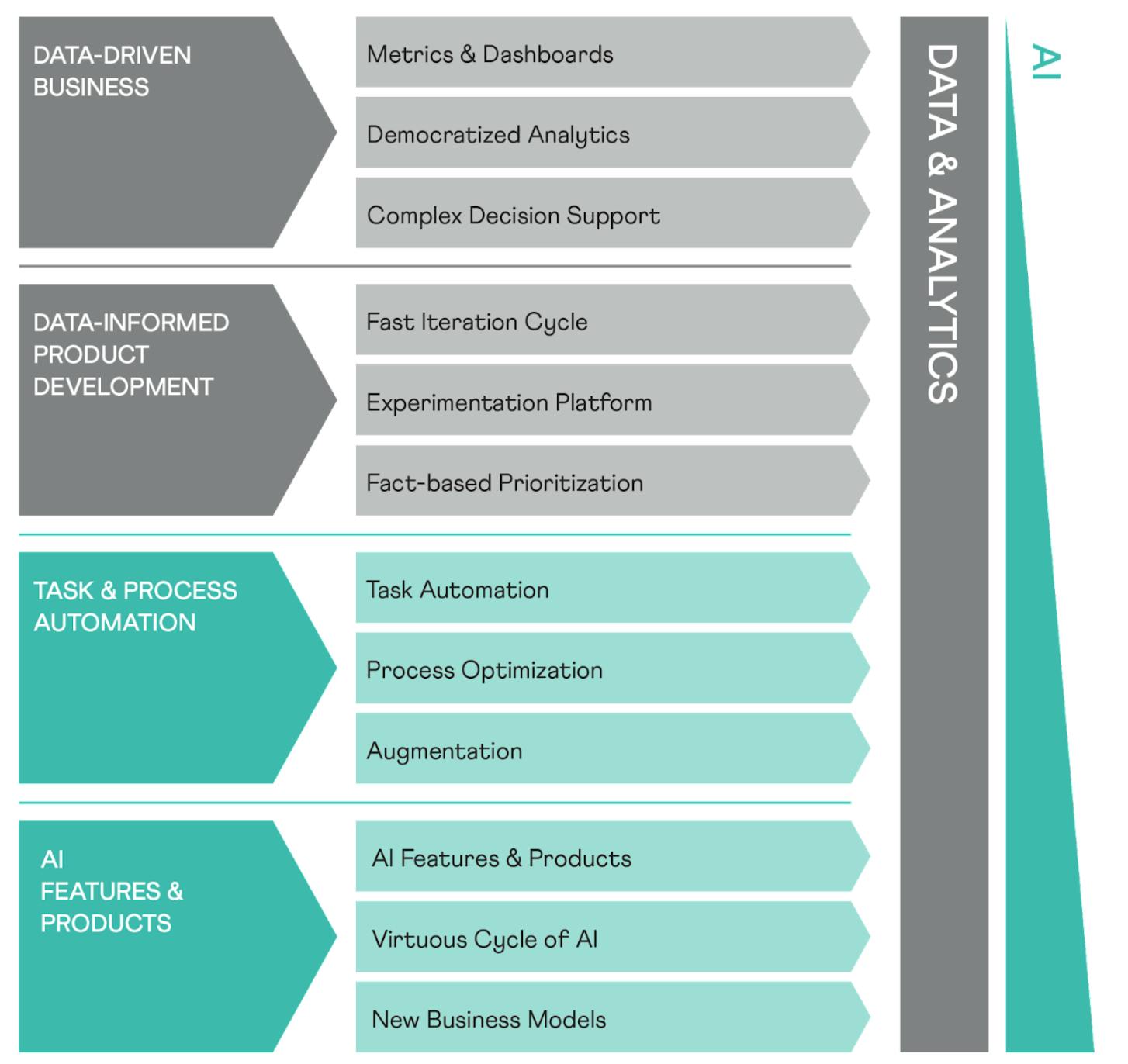
For companies looking to hire data teams, they must first know which of these areas they want help with, and why. This is important in order for the company to be able to prioritize the right profiles and competencies when recruiting. When Galina founded Gradient Descent, a Data and AI consultancy, they spent a lot of time helping organizations in these areas before they could grow and become more data- and AI-enabled.
Who will build things with data?
For candidates applying to open data positions as e.g. Machine Learning Engineers or Data Scientists, it is still often unclear which part of the company they are going to end up in. But once a company has its data use cases and business areas figured out, it can start employing data roles for them. Knowing what specific roles are needed is not always the easiest.
The many different titles and roles of data teams have exploded in recent years. We now have Machine Learning Engineers, Data Scientists, AI Solution Architects, Data Warehouse Engineers, Data Engineers, and Research Scientists, to mention a few. Another example is The Analytics Engineer who was dubbed one of 2022’s top data trends.
Let’s look at a chart showing how different data roles can overlap and divide.
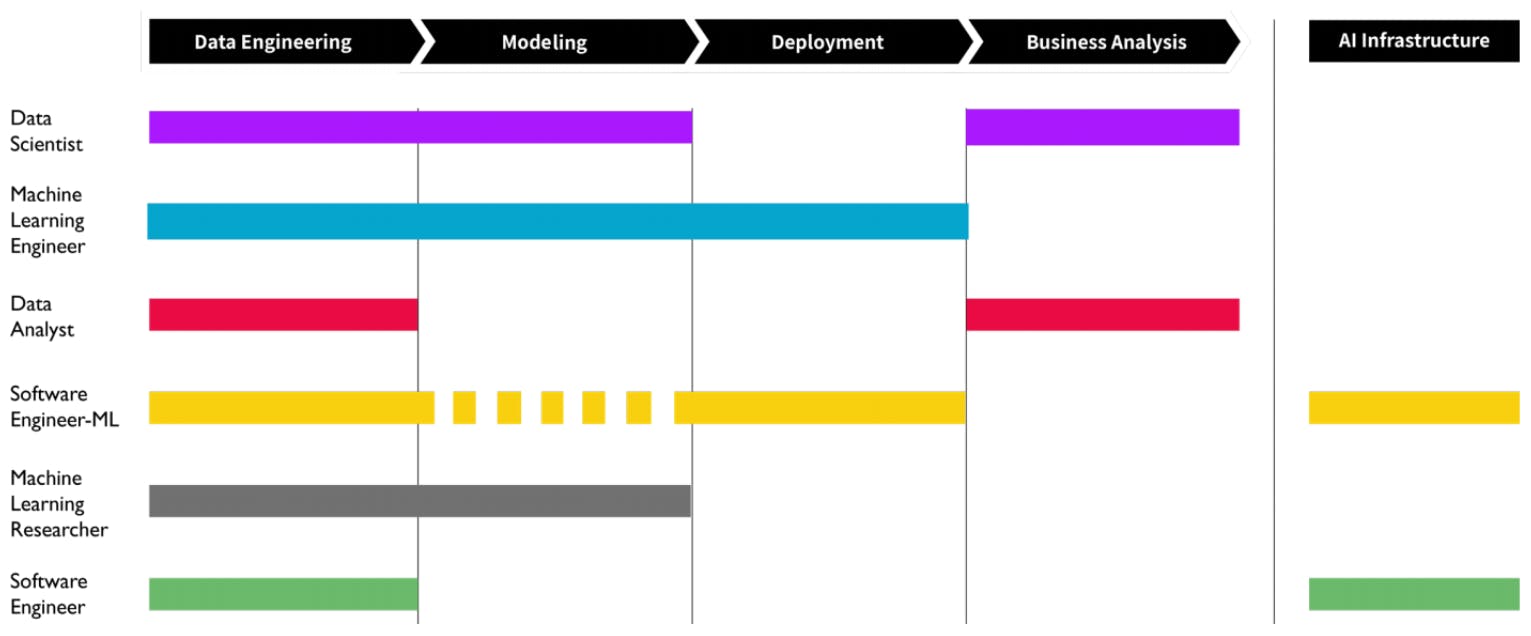
The responsibilities of these roles will often vary between companies and the many titles will likely continue to change and adapt as society’s data capabilities evolve going forward. But by focusing on what you want your data team to build with data, you can make sure to clearly communicate that in your role descriptions and recruitment processes. This will make it much easier to find the right fits for your data team.
Decide on data team composition. After figuring out your data use cases and who will build things with data, the next step is deciding how your data team(s) will work together. In general, there are three different models for this. Centralized, embedded, or hybrid teams.
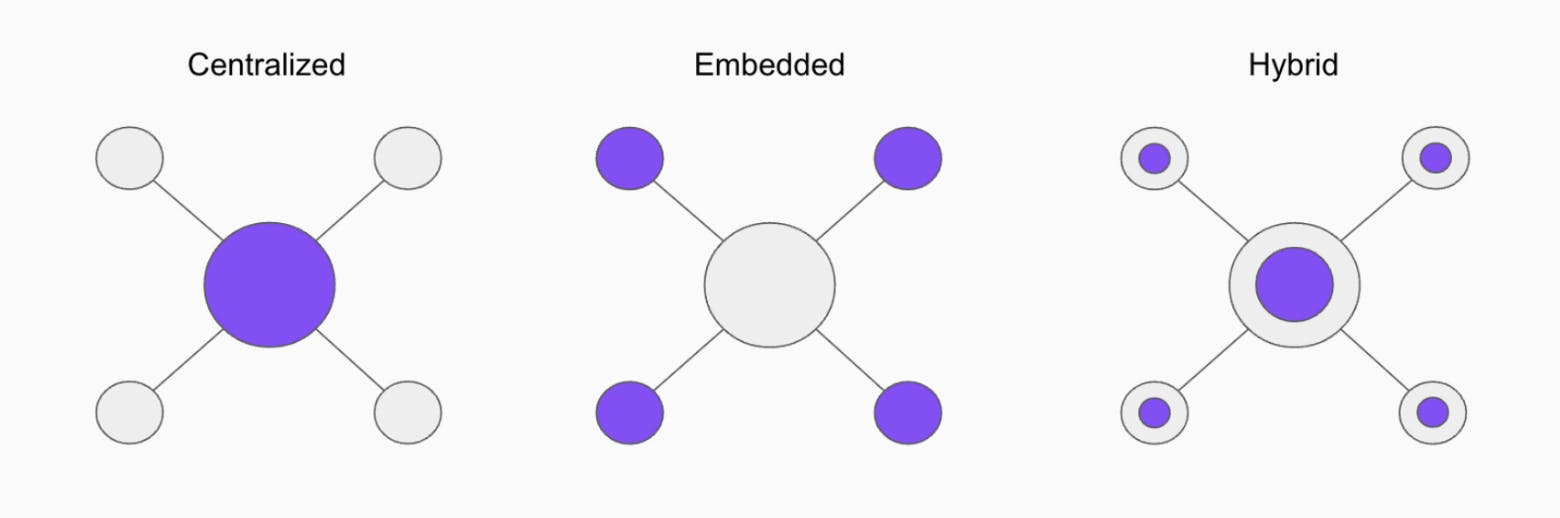
Centralized data teams.
Example: A team of Data Engineers who work centrally on ingesting data sources into a data warehouse.
Embedded data teams
Example: Data Scientists who work fully embedded with separate product teams to help them with their specific business needs.
Hybrid data teams
Ex: A team of Analytics Engineers who work partly on modeling core data tables and partly on analytics embedded in product teams.
There will be pros and cons with each model and ultimately you need to evaluate which one best fits your specific organization and company culture. Although, in recent years, there has been a growing trend for decentralized data teams. One popular strategy making use of such decentralization is the data mesh framework.
Meantime, Galina suggested that perhaps avoiding calling the central team (whether in a centralized or a hybrid setup) “a center of excellence” is best avoided, as it can create unnecessary tensions within any company.
2. Find great candidates and make them arrive happy
To make this easier, it is key to both look deeply within the network that a manager should nurture over the years and engage your recruiting team. For data roles, you will likely want to also engage outside recruiters who are focusing specifically on data roles. The latter is especially important for any type of lead data role, like Head of Data or Analytics Manager.
Galina introduced an old classic from the Rands in Repose blog by Michael Lopp, one of the most experienced engineering managers in the tech industry, who discusses how engineering managers should look at hiring, and how much time they should spend on it (“for every open job on your team, you need to spend one hour a day on recruiting-related activities” - up to 50% of their time!). This short post delves into how the recruiting funnel works and has great simple tips along the length of the funnel: from finding candidates to making sure people don’t just sign the offer, but also arrive on their first day happy.
3. Evaluate candidates using these four steps
When evaluating candidates, you want to achieve consistency and clear communication across the entire interview process–that means before, during, and after the actual interview steps take place.
- Create a list of what skills to evaluate on. This way, different interviewers will be looking for the same skills, and different candidates will be evaluated on the same criteria.
- Ensure a consistent interview process. Standardize as many parts as possible. A list of skills to evaluate on is one way to ensure consistency.
- Communicate clearly–before, during, and after. Clear and reliable communication during all of the interview steps will make the process more enjoyable for the candidates, as well as make your company more attractive to work for. This shows that your company values their time and effort.
- Ensure comprehensive and uniform evaluation. Evaluate all of the basic skills needed for carrying out the job, and don’t only focus on certain elements. Make sure that your evaluation also follows a systematic approach, so that every candidate is evaluated fairly.
Having these steps in place will not only make your recruitment process more consistent and efficient, but it will also make it easier to reproduce the steps across different roles and departments.
Pop quizzes make for consistent and effective evaluation
For data roles, there are surprisingly many candidates who cannot answer basic technical questions related to the role they're applying for, even though their resumes suggest that they would. Screening the candidates with some basic questions can be really useful to filter out unqualified candidates, as well as understand where in the diagram of the many data skills (see above) a candidate lands.
Here are some examples of technical topics to ask about, depending on the role you’re recruiting for:
✔ Basics of databases
✔ Basics of programming
✔ Basics of probability
✔ Basics of machine learning
✔ Basics of data engineering
Example of a basic SQL question
Which query returns the number of unique users in our table books? The table has two fields: user_id and book_id.
- SELECT user_id, COUNT(*) FROM books GROUP BY book_id
- SELECT SUM(distinct user_id) FROM books
- SELECT COUNT(*) FROM books
- SELECT COUNT(distinct user_id) FROM books
Example of a basic Machine Learning question
What is the process of introducing additional variables to the loss function in order to reduce overfitting called?
- Regularization
- Normalization
- Optimization
- Levelling
Tip: For a full guide on how to hire Machine Learning Engineers specifically, check out this guide by Toptal.
4. Close candidates
A good candidate will naturally have a higher likelihood of being picked up by other companies. Closing them fast is equally important for lowering that risk as it is for showing the candidates that you value them. Aim for a short recruitment process for all candidates, regardless if it leads to an offer or not. An optimal duration, from initial contact to the final offer, is less than one month.
The TL;DR
In summary, recruiting data teams efficiently boils down to the following four steps:
1. Figure out what your company needs. What do you want to build with data, and what roles and business functions should build it? There is an abundance of titles for data professionals—focus instead on what needs to be done and what skills it requires.
2. Find candidates by working closely with your recruiting team, external data role recruiters, and, perhaps most importantly, building and maintaining your network.
3. Evaluate candidates using comprehensive and consistent processes. Ask basic technical questions early on to quickly filter out unqualified candidates.
4. Close candidates quickly to be respectful of their time and to avoid losing them to other companies. Always aim for a recruitment process that takes less than a month.
That’s it. Four simple steps to recruit data teams for your organization. Skip the unicorns.
Do you have other thoughts or best practices when recruiting data practitioners? Don’t hesitate to let us know!
Heroes of Data is an initiative by the data community for the data community. We share the stories of everyday data practitioners and showcase the opportunities and challenges that arise daily. Sign up for the Heroes of Data newsletter on Substack and follow Heroes of Data on Linkedin. This article was summarized by Emil Bring, based on a presentation by Galina Esther Shubina at a Heroes of Data meetup in October 2022.