Ever since the advent of cloud during the early 2010s, I’ve heard business executives complain about investments into AI and described them as black holes: a lot of investments go in, but very little business value ever comes out. I’ve seen this result in a more careful mindset among business executives when it comes to everything AI during the last half a decade. However, ever since ChatGPT was introduced to the world on November 30, 2022, the mindset has shifted drastically. Now, once again, everyone is bullish on AI investments.
Based on conversations and collaborations with hundreds of business- and data leaders over the years, I’ve identified three success factors on how to succeed with AI investments: AI-problem fit, prioritization among AI use cases, and a clear strategy for how to manage data debt.
In this blog post, I’ll give an introduction to the three areas, but I recommend anyone who wants the deep dive to check out The Data Leader’s AI Guide.
Finding the best AI use cases—the importance of AI-problem fit
First out of the success factors is finding AI-problem fit.
Each time a new technology is hyped, people have a tendency to apply it to every imaginable problem. AI is no exception. It is not uncommon to hear top management teams mandate their teams to “implement AI”. In other words, they are requesting their teams to apply a specific solution without first specifying the problem. Ideally, the problem definition should happen first. After this, potential solutions can be decided upon. AI is a potential solution to some, but far from all, problems. As a result, AI should not be used to solve all problems.
The first step in identifying AI use cases with AI-problem fit is to specify a prioritized list of problems that the business is facing. This list of problems stems from the actual needs of the underlying business and should not be affected too much by the introduction of new technologies such as AI. The number of potential solutions to each problem on the list might be many and varied, e.g. the redesign and improvement of processes, or the integration and automation of different IT systems. Sometimes, AI turns out to be the best potential solution to a prioritized business problem. Then, and only then, is there an AI-problem fit —and the AI use case is worth looking into further.
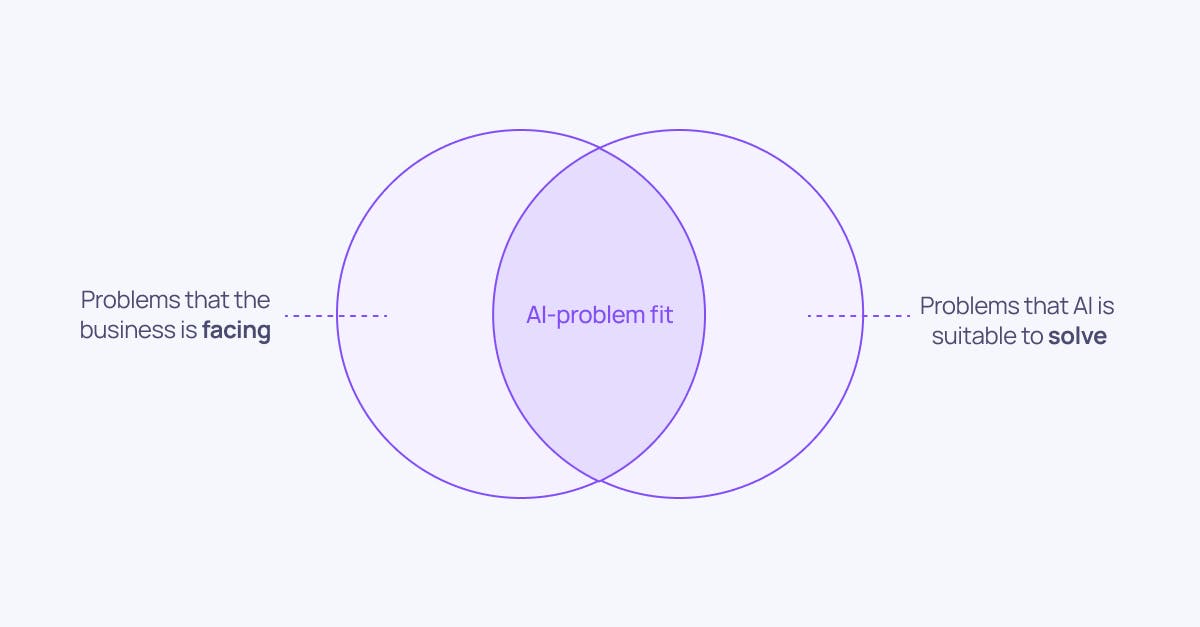
Use cases have an AI-problem fit when there is an overlap between the problems that the business is facing and the problems that AI is a suitable solution to.
Prioritizing among AI use cases
It’s not uncommon to end up with more lucrative AI use cases with strong AI-problem fit than the organization can handle right away. In such situations, it’s important to prioritize among them so that the right use cases go first and create momentum for the rest.
I recommend using the “AI use case prioritization framework” for prioritizing among the AI use cases. When using the framework, data leaders should score each AI use case across the two dimensions of business value and implementation complexity.
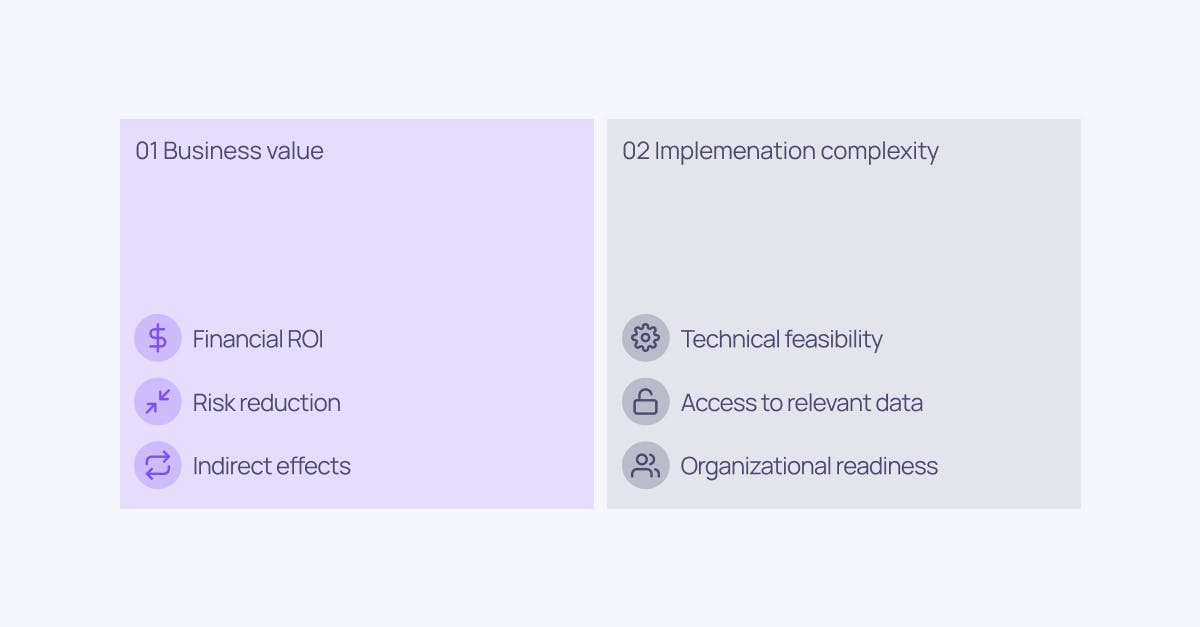
Prioritization of use cases should consider both business value and implementation complexity.
When scoring a use case for business value, three things should be considered:
Similarly, implementation complexity should consider three factors:
Based on these dimensions, I recommend mapping AI use cases onto a 2x2 matrix with business value on the y-axis and the implementation complexity on the x-axis. It’s important to note that a mapping like this is a living document. The business value and implementation complexity of use cases can change over time.
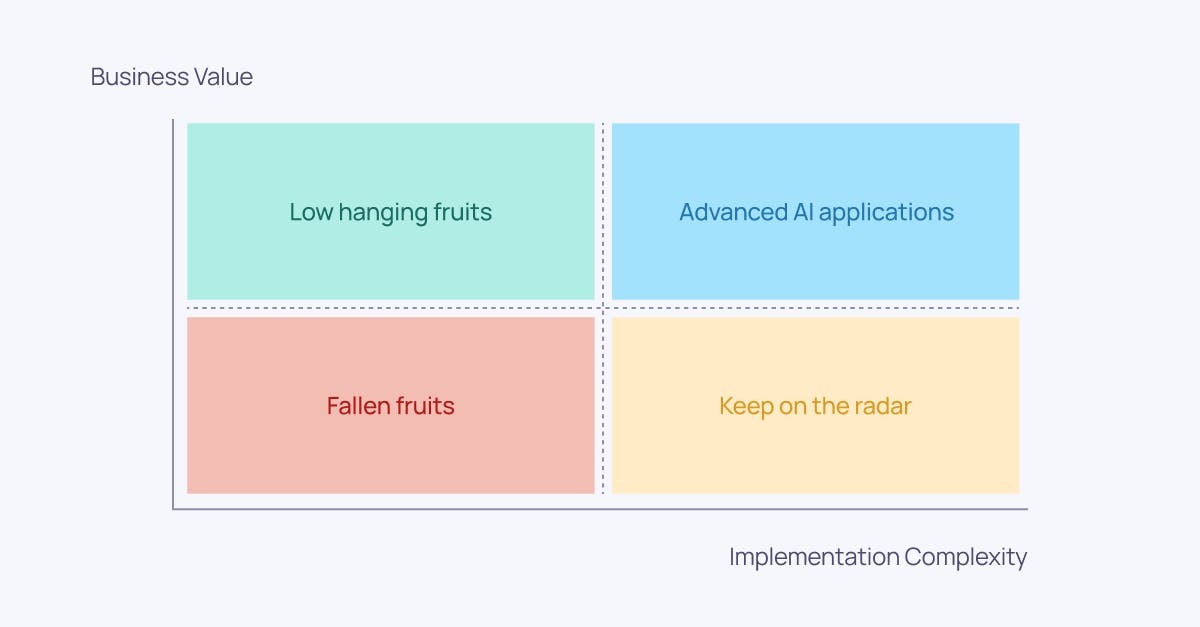
AI use case prioritization framework, which helps companies prioritize their AI use cases based on value to the business and implementation complexity.
For an organization that’s just starting out with AI, I recommend prioritizing use cases in the following order:
- “Low hanging fruits” —have high business value while being low in implementation complexity. This also means they are perfect for creating initial success stories of AI use cases.
- “Fallen fruits”—These use cases entail relatively low risk, but also yield relatively low rewards. It is not uncommon for companies to choose AI use cases that belong to this category when they want to get started with AI, due to the ease of implementation, despite the limited business value. This is especially true if a business cannot identify any clear Low hanging fruits.
- “Advanced AI applications''—while the business value is high for these use cases, the risk is also high when it comes to successful implementations. Therefore, they should be approached only when the Low hanging fruit use cases are exhausted and the organization has gained some momentum and learnings regarding AI use cases.
- “Keep on the radar”—Companies should not act on these use cases, but rather revisit them if implementation complexity decreases or business value increases.
Once the AI use cases have been prioritized, it is time to start executing—if possible. I’m consistently seeing how difficult and time consuming it is to fully put an AI use case in production and get it adopted. Why?
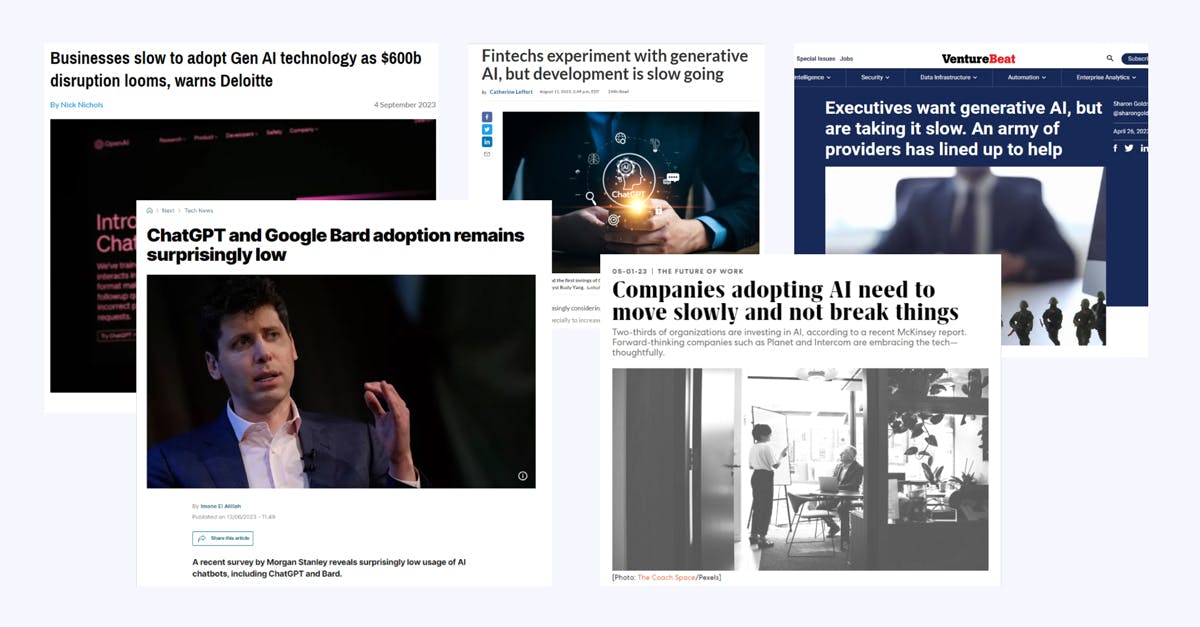
It's more common than not to struggle with data & AI implementation.
Data debt—the biggest bottleneck for AI adoption
A large amount of accumulated data debt is the single most prevalent reason why I see companies struggling to implement AI use cases at the desired speed. This is because data debt is the most prominent factor that drives implementation complexity of AI use cases. For example, many data scientists spend 60-80% of their time cleaning & organizing data which means the technical feasibility of the use case is low. We’ll soon have a look at why this is an example of data debt, but I’ll first define the term:
Data debt is the build up of data-related problems over time that ultimately lowers the return on data investments. It functions like a tax—the more data debt you have accrued, the higher the tax rate.
For many organizations, the tax rate on the data investments is close to 100%, which is why they rarely see any positive ROI at all on their data investments. There are three different types of data debt: data-related, technology-related and people & process-related.
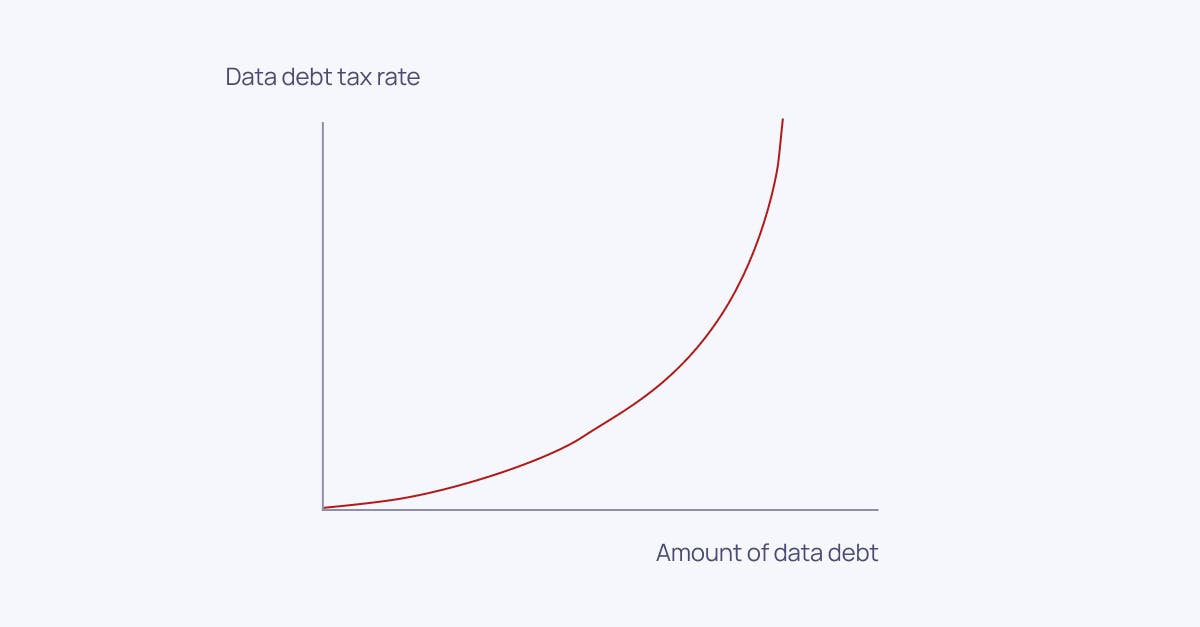
The data debt tax rate increases with an increased amount of data debt.
Data debt comes in three different forms: data-related-, technology-related-, and people & process-related data debt. Next, I'll briefly cover these types of data debt, but for more in-depth information, I recommend this blog post.
Data-related data debt
Data-related data debt is often the most critically felt type of data debt since it impacts the actual data. It includes things like poor data quality, “dark data” that sits in data warehouses without being used, and data silos that make analytics challenging or impossible. A study in Harvard Business Review showed that only 3% of companies’ data meet basic quality standards. In other words, 97% of data among corporations is of poor quality and cannot be used in business critical use cases. Poor data quality is estimated to cost companies several trillions USD per year in the US alone and severely limits an organization’s ability to quickly launch AI use cases.
Technology-related data debt
Technology-related data debt includes problems like fragmented data stacks with too many tools, inadequate tooling for proper governance, limited scalability, and a poor fit for self-service analytics. Technology-related data debt makes it difficult to get return on data investments since inappropriate tool setups are hard or impossible to work with for data- and business teams alike. They limit companies’ capabilities to scale AI use cases in a cost-efficient manner or fulfill regulatory requirements on AI and data.
People & process-related data debt
This type of debt includes low organizational trust in data, poor alignment between business- and data teams, a culture that’s not data-driven, and lack of ownership in relation to fixing data quality issues. People & process-related data debt makes any new AI initiative significantly more difficult to launch. It requires large investments in change management for the slightest bit of progress in bringing alignment between various stakeholders involved in implementing the AI use case.
Paying back data debt with the Data Trust Workflow
Given that the management of data debt is the single most important factor for determining the success of an AI investment, it should be at the top of the agenda for every company that wants to invest in, and succeed with AI.
In the era of data and AI, data debt is no longer something that only the data team cares about. It is something that business teams and data teams need to actively collaborate on. Business teams need to educate themselves on what it means to work with data, and data teams need to educate themselves on what is important for the business. The management team needs to have a plan for managing data debt, in the same way they have a plan to manage financial debt, technical debt and organizational debt of the company.
There is a proven methodology I recommend companies follow to manage and pay back data debt: the Data Trust Workflow. It’s comprised of three steps:
- Prioritization of all data assets based on their impact on the business
- Validation of the prioritized data to ensure its quality
- Improvement of the prioritized data through initiatives to pay back data debt across the data-, technology, and people & process dimensions.
This process should be repeated continuously over time as additional use cases of data and AI are added and/or changed.
In conclusion
To succeed with AI, it’s important to identify use cases with AI-problem fit that creates business value while being suitable for AI technology—not everything should be solved with AI. Among the identified AI use cases, it is important to prioritize which ones to do first in order to get off to a good start, as the successful implementation of AI use cases can help to build good momentum through a lot of good learnings and increased cultural acceptance of AI use cases within the organization. Last but not least, it is of the highest importance to properly manage data debt through the Data Trust Workflow, since data is the food for AI.
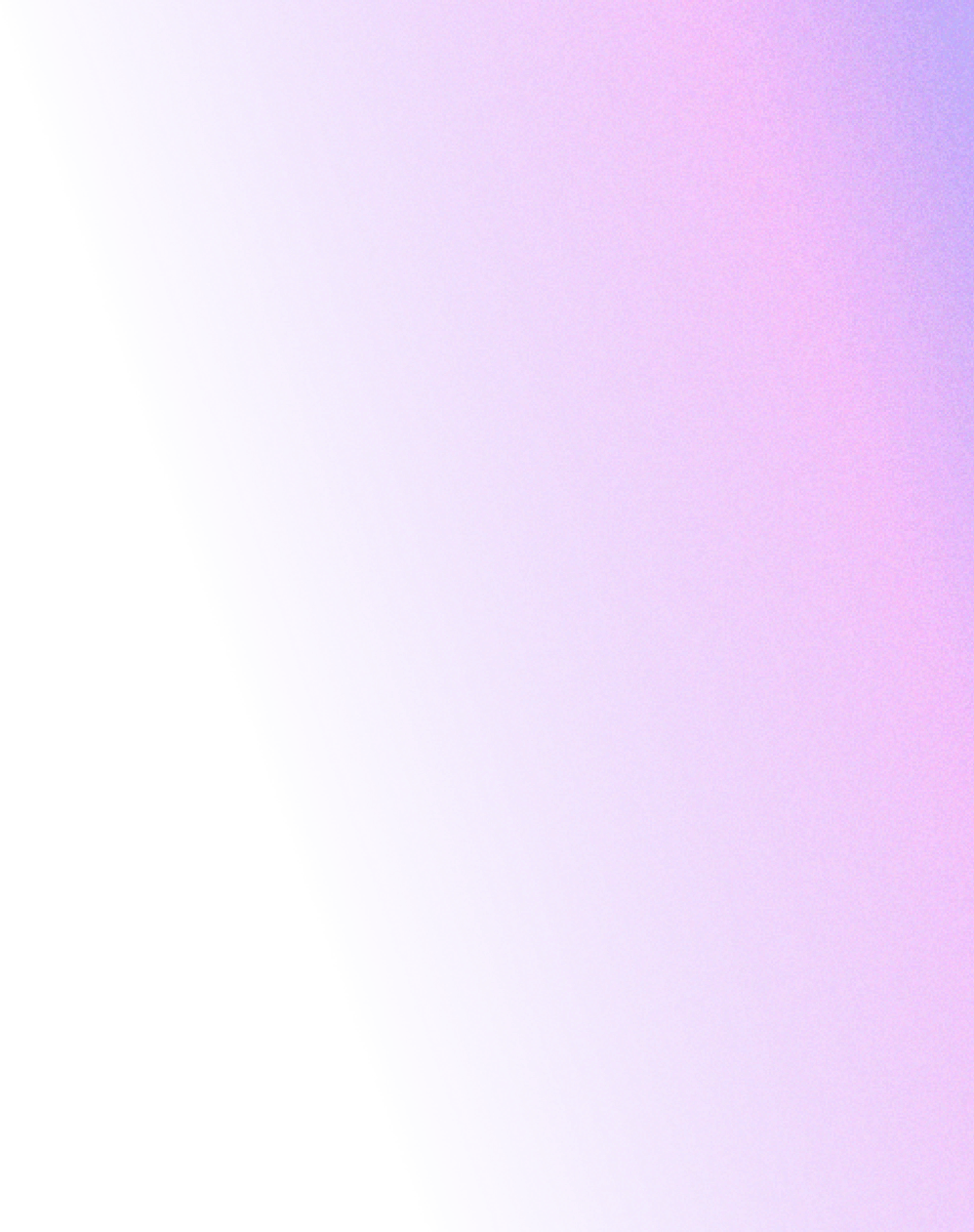
Are you ready for AI but your data isn't?
Get in touch