Ever since ChatGPT was introduced to the world on November 30, 2022, everyone became bullish on AI investments. However, data leaders trying to implement AI and get return on data investments will have a tough time. They’re crippled by data debt that makes launching new data & AI use cases challenging at best and impossible at worst. Validio’s Data Trust Platform is built specifically to combat data debt, and so I thought it would be fitting to explain data debt in more detail, and why it’s so detrimental to data leader’s efforts to deploy AI and get return on their data investments.
What is data debt?
Data debt is the build up of data-related problems over time that ultimately lowers the return on data investments. It functions like a tax—the more data debt you have accrued, the higher the tax rate. For many organizations, the tax rate on data investments is close to 100%, which is why they rarely see any positive return on their data investments.
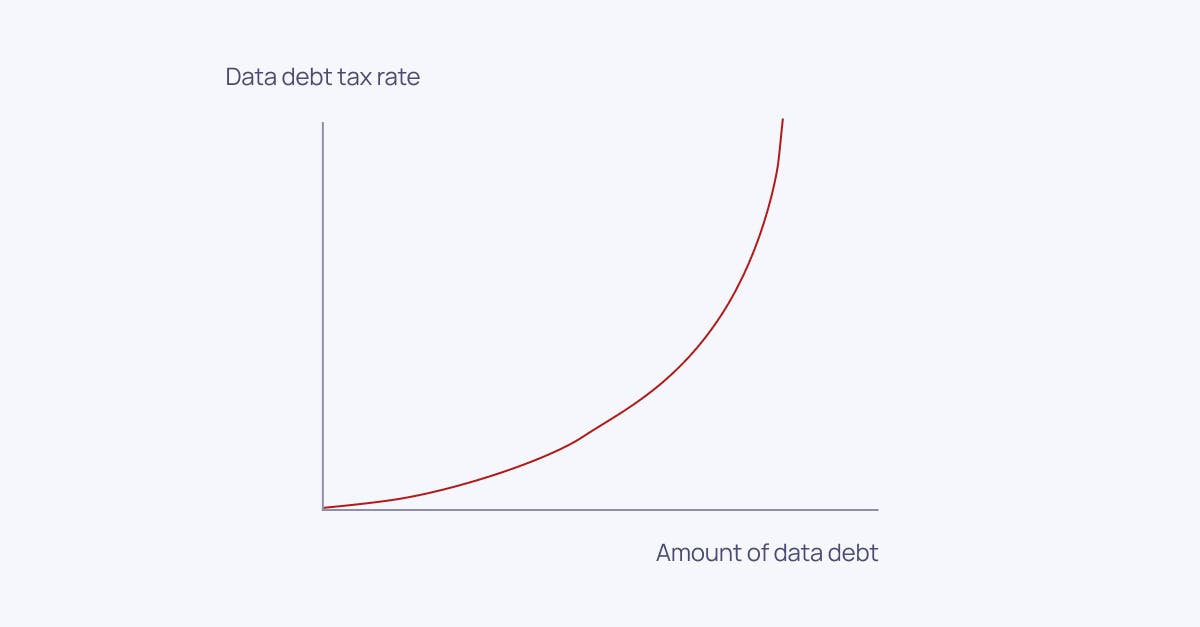
The data debt tax rate increases with an increased amount of data debt.
There are three different types of data debt: data-related, technology-related and people & process-related. Let's take a closer look at each type.
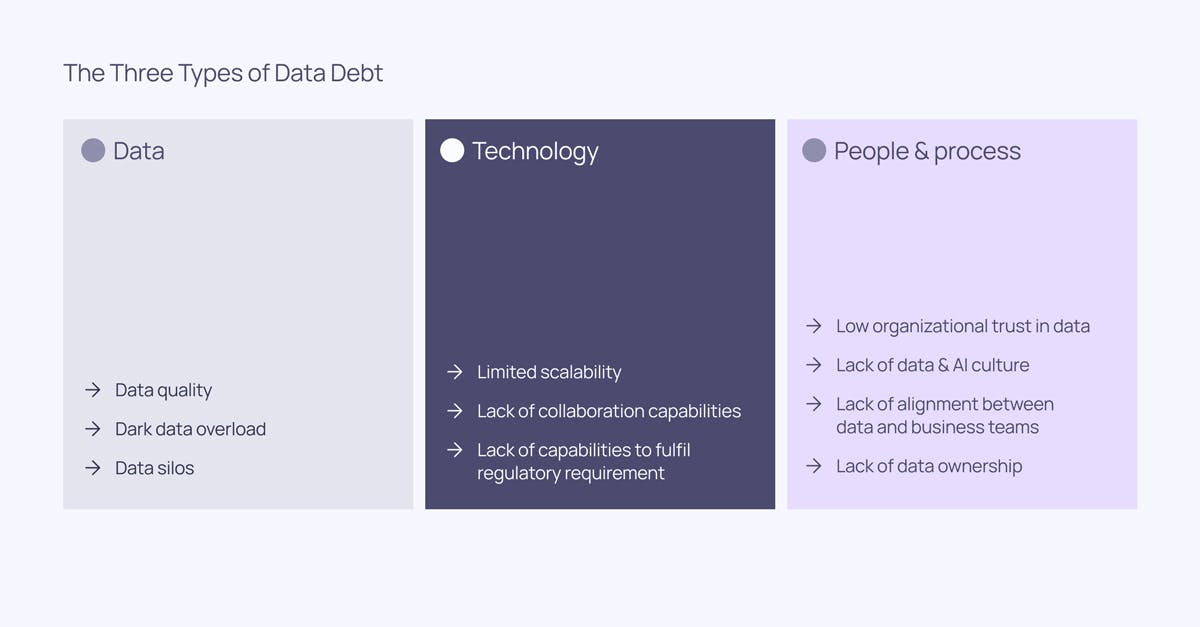
There are three different types of data debt: data-related, technology-related and people & process-related.
Type 1: Data-related data debt
Data-related data debt makes it hard to get return on data investments since it makes data untrustworthy and/or not usable for high-stakes use cases. This can manifest in a number of ways, including poor data quality, dark data overload, and data silos.
Poor data quality
A study in Harvard Business Review showed that only 3% of companies’ data meet basic quality standards. In other words, 97% of data among corporations is of poor quality and cannot be used in business critical use cases. Poor data quality is estimated to cost companies several trillions USD per year in the US alone. Despite this, most companies have not prioritized the problem of poor data quality as much as they should. In the light of Generative AI, that’s starting to change. The State of Analytics Engineering report from 2023 showed that data quality and observability is the most popular area of future investment, with almost half of the respondents planning to invest in the area.
Andrew Ng (Founder of Google Brain, former Associate Professor at Stanford) agrees, and has started to evangelize what he calls a data-centric approach to AI where you shift your focus towards managing data debt rather than improving your AI algorithms. “The real differentiator between businesses that are successful at AI and those that aren’t, is down to data: What data is used to train the algorithm, how it is gathered and processed, and how it is governed? /…/ “the shift to data-centric AI.” is the most important shift businesses need to make today to take full advantage of AI,” Ng argues.
Dark data overload
The amount of data that is being generated and collected is growing exponentially. For many companies, as little as 10% of all that data is relevant and actually used for business critical purposes. The rest, 90% of the data, never sees the light of day again after being ingested and stored, which is why it is called “dark data”. This is problematic for several reasons. Firstly, dark data drives a big amount of the cost that goes into data and AI investments, but since it is never being used it provides no return on investment. Secondly, even though dark data is not being used, it dilutes focus and attention from the data that actually matters. For example, data management efforts, such as GDPR compliance work, need to include the dark data even if it is never being used for any specific use cases.
Data silos
The modern data stack, which consists of a big number of best-of-breed tools, has made it easy and relatively cheap for companies to get up and running with a state-of-the-art data stack. A flipside is that it has resulted in a siloed data stack with different solutions for data integration, data streams, data lakes, data warehouses, data visualization, data transformation, data orchestration, data cataloging, data lineage, data observability, etc. It is difficult for companies to work efficiently and get a complete overview of their data as a result—data ends up being siloed in different parts of the data stack. Matt Turck has provided a mapping of the modern data stack, clearly showing how the modern data stack has exploded in complexity and number of components.
Type 2: Technology-related data debt
Technology-related data debt makes it difficult to get return on data investments since inappropriate tool setups are hard or impossible to work with for data- and business teams alike. It might also limit companies’ capabilities to scale AI use cases in a cost-efficient manner or fulfill regulatory requirements on AI and data. It can show up like limited scalability, a lack of collaboration capabilities, or a lack of capabilities to fulfill regulatory requirements.
Limited scalability
The scalability that is required of the data stack depends on the AI use case. Some AI use cases require model inferences to be made in real-time or near real-time, which means that the data stack needs to support real-time streaming for these use cases to even be considered. Some AI use cases might deal with large data volumes, such as several billions of data points that need to be processed every three hours. The data stack in place needs to be able to scale from a pure data processing, storing and latency point of view. It needs to also scale cost-wise, otherwise the ROI of the AI use case will be jeopardized.
Lack of collaboration capabilities
In order to really make data fit for purpose for business critical AI use cases, it is important that data and business teams work together. This usually requires tools that have both a code interface for technical stakeholders as well as a graphical user interface for business stakeholders. Data tech stacks that are heavily skewed towards only code interfaces or graphical user interfaces risk alienating either the business or data teams who are important for the AI use case implementation.
Lack of capabilities to fulfil regulatory requirements
AI is one of the most popular areas when it comes to regulation these days. For example, a deal on the European AI Act was recently reached by the European Union, which puts comprehensive requirements on all so-called high risk AI use cases. One of the requirements is the monitoring of all input and output data that go into AI systems for high risk use cases. Most companies right now have limited to no capabilities in place to monitor the input and output data in a manner that fulfills the regulatory requirements, both when it comes to the granularity, scale and frequency that’s required. Many lucrative AI use cases will be hindered until companies have procured and developed the capabilities to fulfill the regulations. The cost of non-compliance is associated with fines up to 35 million Euro or 7% of global turnover.
Type 3: People & process-related data debt
People & process-related data debt makes any new data initiative significantly more difficult to launch. It requires large investments in change management for the slightest bit of progress in bringing alignment between various stakeholders involved in implementing the AI use case. Examples include low organizational trust in data, lack of data & AI culture, lack of alignment between data & business teams, and a lack of data ownership.
Low organizational trust in data
A common assumption among companies is that the data they have collected is of high quality. Therefore, companies who are early in their data and AI journey are often the ones who have the lowest trust in their data, since they have just started to realize how poor the quality is. This realization often results in a halt to all data and AI use cases, and people within the organization revert back to making decisions based on “gut feel”.
Lack of data and AI culture
Data and AI is often met with a lot of skepticism within organizations. People are afraid of being heavily affected by the introduction of AI use cases in their day-to-day work, since AI is often used to automate and improve existing processes and workflows. Some people even fear losing their jobs. Without the buy-in from business stakeholders, the identification of use cases with AI-problem fit is being hindered.
Lack of alignment between data and business teams
Most companies today struggle with the alignment between data teams and business teams. It’s not uncommon for companies to organize their data professionals into a “central data platform team” which should enable the rest of the organization to use data and AI. The missing link is oftentimes the actual processes to ensure that there is an alignment between what the different business units need and what the central data platform team ends up providing. Without good processes in place, it is very common that the central data platform teams end up building modern and state-of-the-art data platforms with little to no actual business use cases in mind. It goes without saying that it is difficult to get business impact and return on your data investments with such a setup.
Lack of data ownership
It’s a common expectation within companies that the data team should be responsible for providing everything from the raw data to the AI models that will be put in production. If things go wrong, the data team is to blame. However, in reality, there are many different stakeholders involved in the data journey. The data team is oftentimes not even involved in the majority of the data journey. Therefore, it’s not reasonable that they should own the data, including all of the data quality issues, all the way from data ingestion to consumption. For example, there are data producers (e.g. software engineers or owners of different products/systems that generate data), data transporters (e.g. data engineers and data platform teams who provide the data infrastructure to move data from point A to point B) and data consumers (e.g. analytics engineers, data scientists, analysts or business stakeholders who are the end consumers of the data). All of the different stakeholders are responsible for different parts of the data journey, all of which can end up introducing data quality issues. For example, if a data producer changes the API of a website all of a sudden, that might have an impact on the ingestion of the data. Data transporters might build data infrastructure that transports data once a day, meaning that data will not be fresh more than once a day. If a use case requires data more frequently than that, the use case will not be viable. Data consumers often perform a lot of transformations on the raw data to shape it into more usable formats, including joining several data tables. This is a part of the data journey that is highly likely to introduce data quality issues. To distribute the ownership of the data from the data team to all involved stakeholders throughout the entire data journey is a big challenge for many organizations.
How to overcome data debt?
I’ve now covered the three types of data debt, and the future might look bleak. Will we ever be able to effectively pay back data debt? The answer is a strong yes, but it requires thought-through methodology and specific, coherent tooling.
There is a proven methodology I recommend companies follow to manage and pay back data debt: the Data Trust Workflow. It’s comprised of three steps:
- Prioritization of all data assets based on their impact on the business
- Validation of the prioritized data to ensure its quality
- Improvement of the prioritized data through initiatives to pay back data debt across the data-, technology, and people & process dimensions.
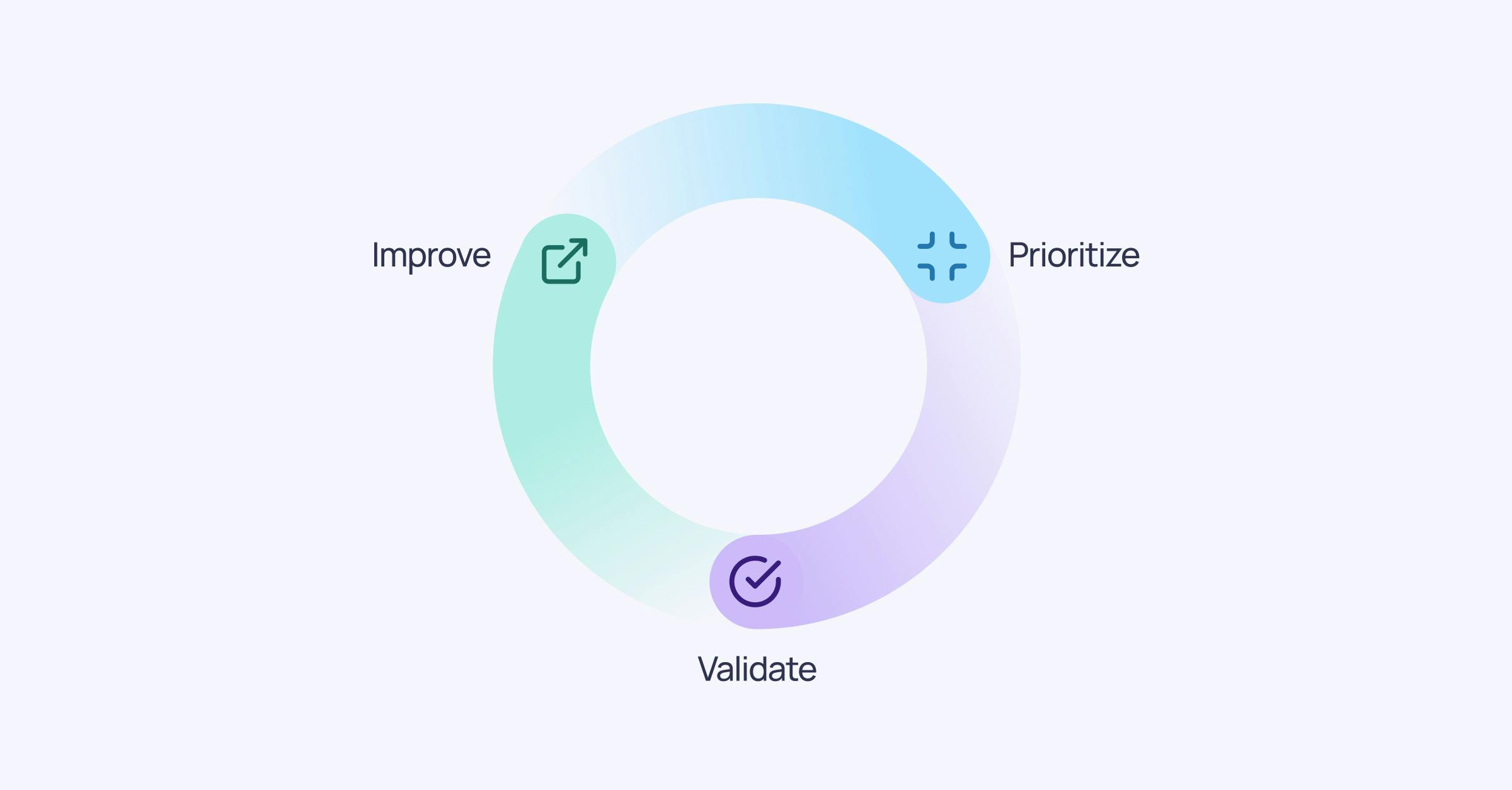
The Data Trust Workflow is a simple and proven methodology to pay back data debt.
This process should be repeated continuously over time as additional use cases of data and AI are added and/or changed. For anyone that’s interested in staying ahead and having a positive impact on the data debt at their companies, I recommend downloading and reading the Data Trust Guide.
In conclusion
Given that the management of data debt is the single most important factor for determining the success of an AI investment, it should be at the top of the agenda for every company that wants to invest in, and succeed with AI.
Data debt is no longer something that only the data team cares about. It is something that business teams and data teams need to actively collaborate on. Business teams need to educate themselves on what it means to work with data, and data teams need to educate themselves on what is important for the business. The management team needs to have a plan for managing data debt, in the same way they have a plan to manage financial debt, technical debt and organizational debt of the company.
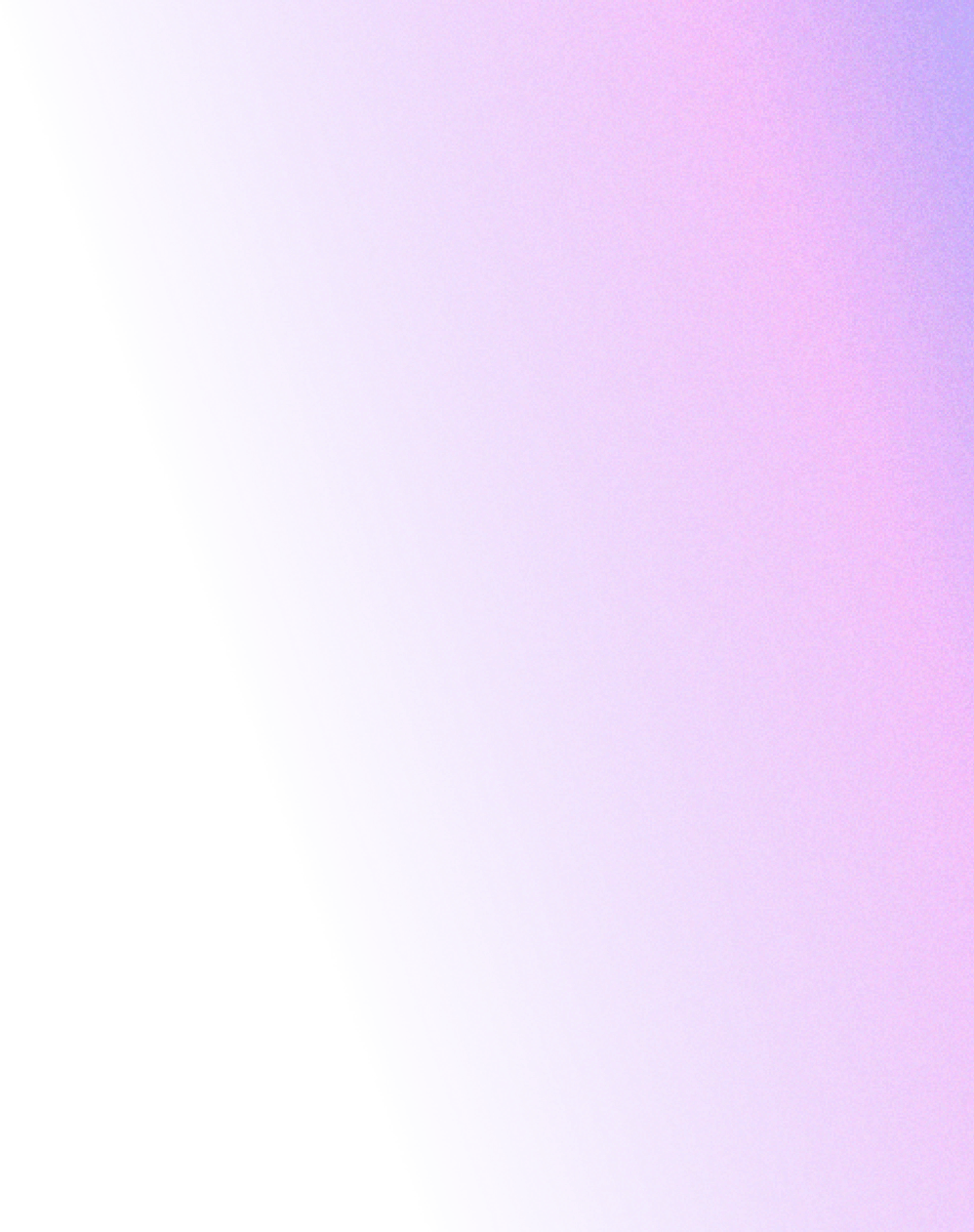
Ready to pay back data debt?
Let's talk