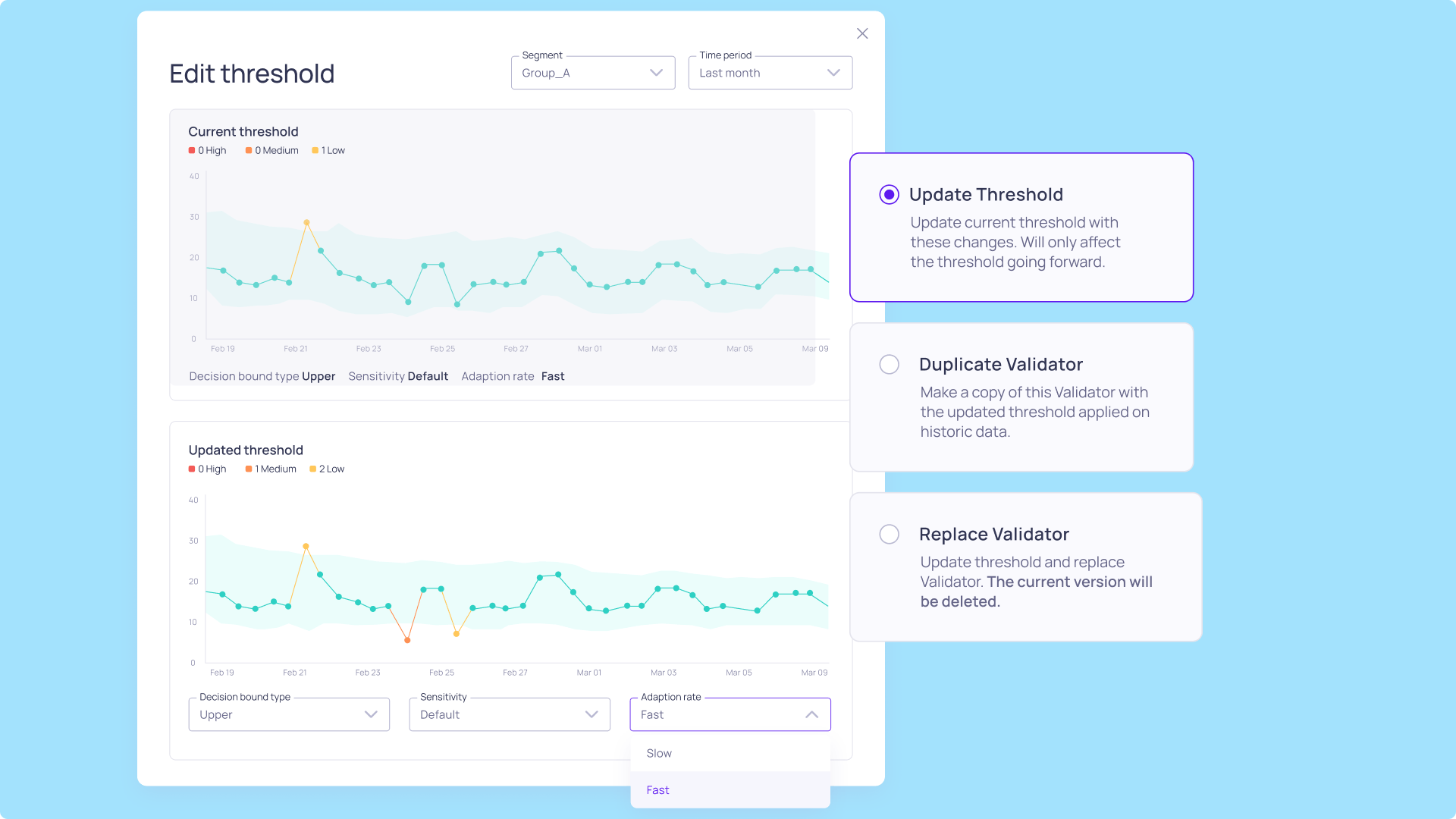
6 min read
Stay on top of your data and metrics with AI-powered monitoring.
Automated anomaly detection
AI-powered monitoring that learns from your data trends to reveal anomalies anywhere in your data stack.
Instant alerts
Bad things happen. But it's good to know when then occur. Validio instantly alerts you about deviations.
Complete lineage
Validio’s root cause analysis shows upstreams and downstreams impact of issues. Resolution has never been easier.
Join the data-led companies that trust Validio with their data quality.
"No matter how good ML models we have, the actions from them don't make sense if the data quality is not there."
David Feldell
Co-founder
“The biggest strength of Validio is the ease of measuring data quality and detecting data anomalies over a wide range of dimensions.”
Anil Sharma
VP, Head of Data Science and Analytics
"Validio helps us feel secure that the data we use is correct. If it’s not correct, we’ll know right away and can understand what was wrong."
David Tran
Data Engineer
Guaranteed reliable data.
Everywhere you need it.
Don’t let any incident go unnoticed
And if in some incredible instance you have a tool we don’t work with - just ask and we’ll build it for you.
Validio is built with security and compliance as cornerstones, to help you stay on top of it.
Your data. Your control.
Validio optimizes metric queries and aggregation, minimizing raw data reads. No data is stored post-processing.
Fully compliant. For your safety and ours.
ISO 27001 & SOC 2 Certified: Our processes and application adhere to industry best practices.
We’ll host or you can. You decide.
Validio offers a self-hosted deployment option in customers Virtual Private Cloud (VPC).
Stay ahead of regulations
Regulations like the EU AI Act will mandate companies to comply. We make sure your data is fit for it.
6 min read
4 min read
5 min read
Sleep well at night
knowing your data is right